The image annotation services market is rapidly growing with AI adoption in areas like autonomous vehicles and healthcare. Accurate, labeled data is essential for reliable AI performance, driving demand for scalable and precise annotation tools.
Staying informed on market trends helps you make smarter decisions, whether using image annotation tools in-house or outsourcing projects.
Key Image Annotation Market Drivers
The rapid growth of the image annotation services market is driven by increasing demand for efficient, high-quality annotation processes across industries. Here are the main factors shaping this market:
AI-Powered Applications
AI applications in autonomous vehicles, healthcare, and retail heavily rely on labeled datasets:
- Autonomous Vehicles: Object detection, lane recognition, and pedestrian identification.
- Healthcare: Tumor detection and diagnostics in medical imaging.
- Retail: Product tagging, visual search, and recommendation systems.
The need for image annotation tools that handle complex, domain-specific tasks is accelerating.
Scalable Solutions for Larger Datasets
As datasets grow, scalable solutions are essential. Businesses turn to:
- Automated Tools: Faster workflows with consistent results.
- Hybrid Approaches: Combining manual expertise with AI for greater accuracy.
Automation and Innovation
Automation in annotation tools streamlines workflows and boosts efficiency. Key innovations include:
- AI-driven feedback for improved accuracy.
- Customizable workflows for specific industry needs.
To keep up with these advancements, businesses must stay informed about the latest developments in image annotation services and tools.
Evolving Industry Demands
Different industries require tailored annotation approaches:
- Defense and Surveillance: Precise annotations for object recognition in aerial or satellite imagery.
- E-commerce: Product recognition and tagging for enhanced customer experiences.
- Urban Planning: Annotated geospatial data for AI-driven infrastructure development.
Emerging Technologies in Image Annotation
Technological advancements are reshaping the way we annotate images for AI, improving efficiency and accuracy for businesses.
AI-Assisted Annotation Tools
AI-powered tools automate repetitive tasks while maintaining accuracy through:
- Pre-Annotation: Automatically generate initial labels for faster workflows.
- Continuous Learning: Adapt to feedback, improving over time.
These innovations save time and reduce costs, letting teams focus on refining models.
Cloud-Based Platforms
Cloud-based solutions simplify annotation with:
- Real-Time Collaboration: Teams can annotate data from anywhere.
- Scalable Infrastructure: Handle growing datasets without on-premises hardware.
- Data Security: Built-in encryption and access controls safeguard sensitive data.
Synthetic Data Integration
Synthetic data complements real-world annotations by:
- Augmenting Datasets: Covering rare or edge-case scenarios.
- Reducing Costs: Lowering reliance on manual annotation.
- Accelerating Iteration: Quickly generating training data for improved accuracy.
Streamlined Workflows
Modern tools enhance workflows with:
- User-Friendly Interfaces: Simplify processes for non-technical users.
- Real-Time Feedback: Ensure quality during annotation.
- Tool Integration: Sync directly with AI training pipelines.
These innovations drive efficiency and scalability across annotation projects.
Outsourcing vs. In-House Image Annotation
Deciding between outsourcing and in-house annotation depends on your goals and available resources.
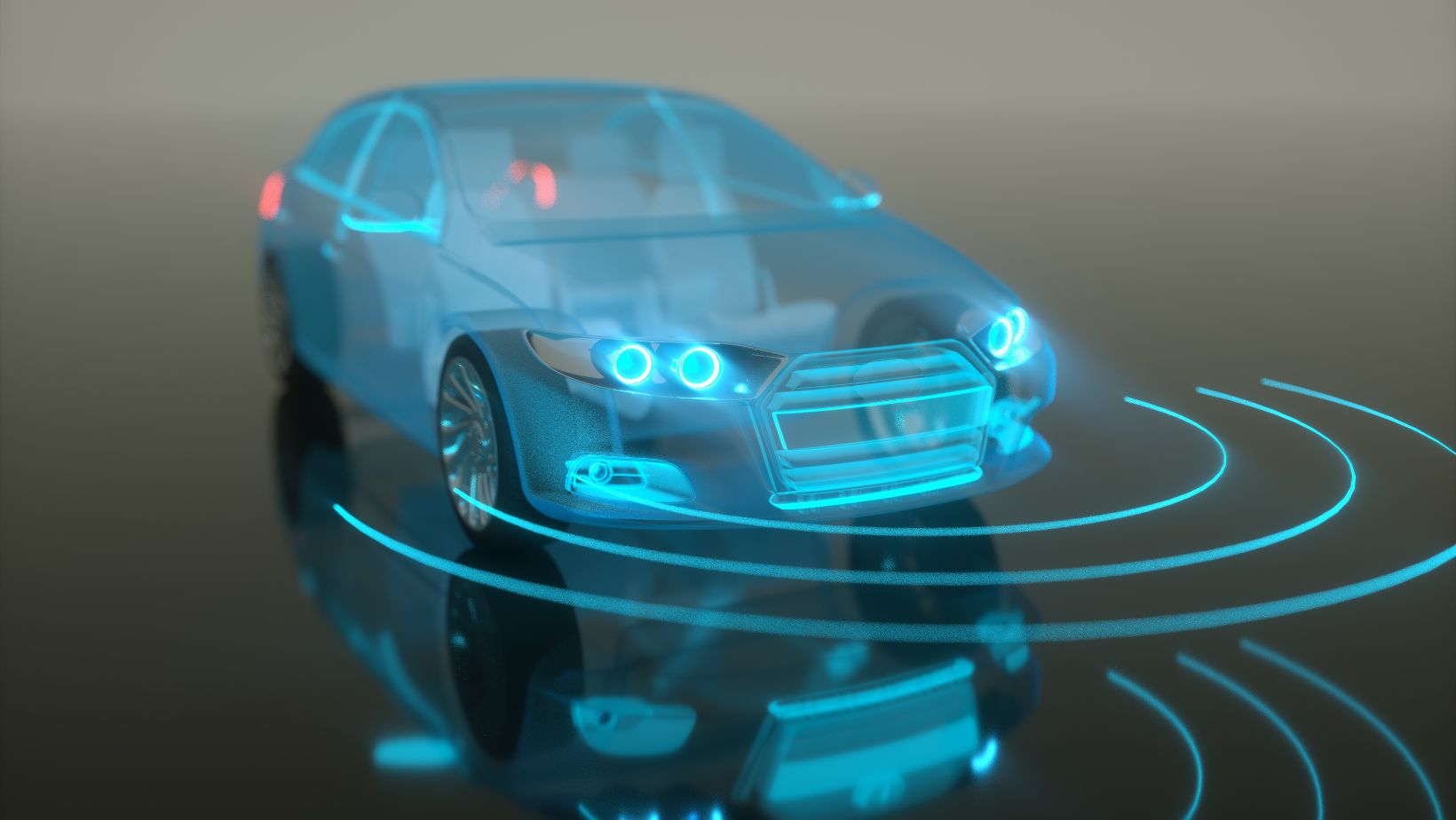
Criteria | Outsourcing | In-House Annotation |
Cost | Lower upfront costs, no hiring or training needed | High initial investment in staff and tools |
Scalability | Easy to scale up or down as needed | Limited by internal team capacity |
Expertise | Access to skilled professionals | Develop specialized in-house skills |
Data Security | Requires strong privacy measures | Full control over sensitive data |
Speed | Quick start with ready workflows | Slower due to setup and training |
Best For | Short-term or flexible workloads | Long-term, consistent projects |
Challenges | Potential communication and privacy issues | High costs and ongoing maintenance |
A hybrid approach blends outsourcing for scalability with in-house handling of sensitive tasks. This balance provides both flexibility and efficiency, making it a practical choice for many businesses.
Industry-Specific Trends
The need for scalable, precise image annotation varies by industry, driving innovation as AI adoption grows.
Healthcare
In healthcare, image annotation plays a critical role in training AI systems for diagnostics and treatment planning. Key applications include:
- Medical Imaging: Annotating X-rays, MRIs, and CT scans for disease detection (e.g., tumors or fractures).
- Pathology: Training AI to analyze slides and identify anomalies in tissue samples.
- Surgical Assistance: Labeling anatomical features to guide robotic surgeries.
The demand for accurate image annotation tools in healthcare continues to grow, with strict requirements for precision and compliance.
Autonomous Vehicles
Autonomous vehicles rely heavily on annotated datasets to understand and navigate their environment. Applications include:
- Object Detection: Identifying pedestrians, vehicles, and obstacles in real time.
- Lane Marking Recognition: Annotating road lanes for safe navigation.
- Traffic Sign Identification: Teaching AI to detect and understand signs and signals.
Annotate images for AI with high-quality annotations to ensure safety and reliability in this industry.
Retail and E-Commerce
Retail and e-commerce businesses use AI-powered systems to improve customer experiences. Annotation tasks focus on:
- Product Tagging: Annotating product images for better searchability and categorization.
- Visual Search: Training AI to match uploaded images with similar products.
- Recommendation Systems: Enhancing personalization by analyzing labeled customer preferences.
Efficient annotation workflows allow businesses to adapt quickly to changing consumer trends.
Urban Planning and Infrastructure
Geospatial data annotation supports urban planning efforts through:
- Satellite Image Analysis: Identifying land use patterns and natural features.
- Infrastructure Mapping: Annotating images for AI to assess and plan construction projects.
- Traffic Monitoring: Using labeled data to improve traffic flow and reduce congestion.
These applications rely on precise annotations to ensure accurate AI predictions and insights.
Ethical and Privacy Considerations
As image annotation services grow, privacy and ethics matter more than ever. Handling data responsibly is key to keeping trust and following the rules.
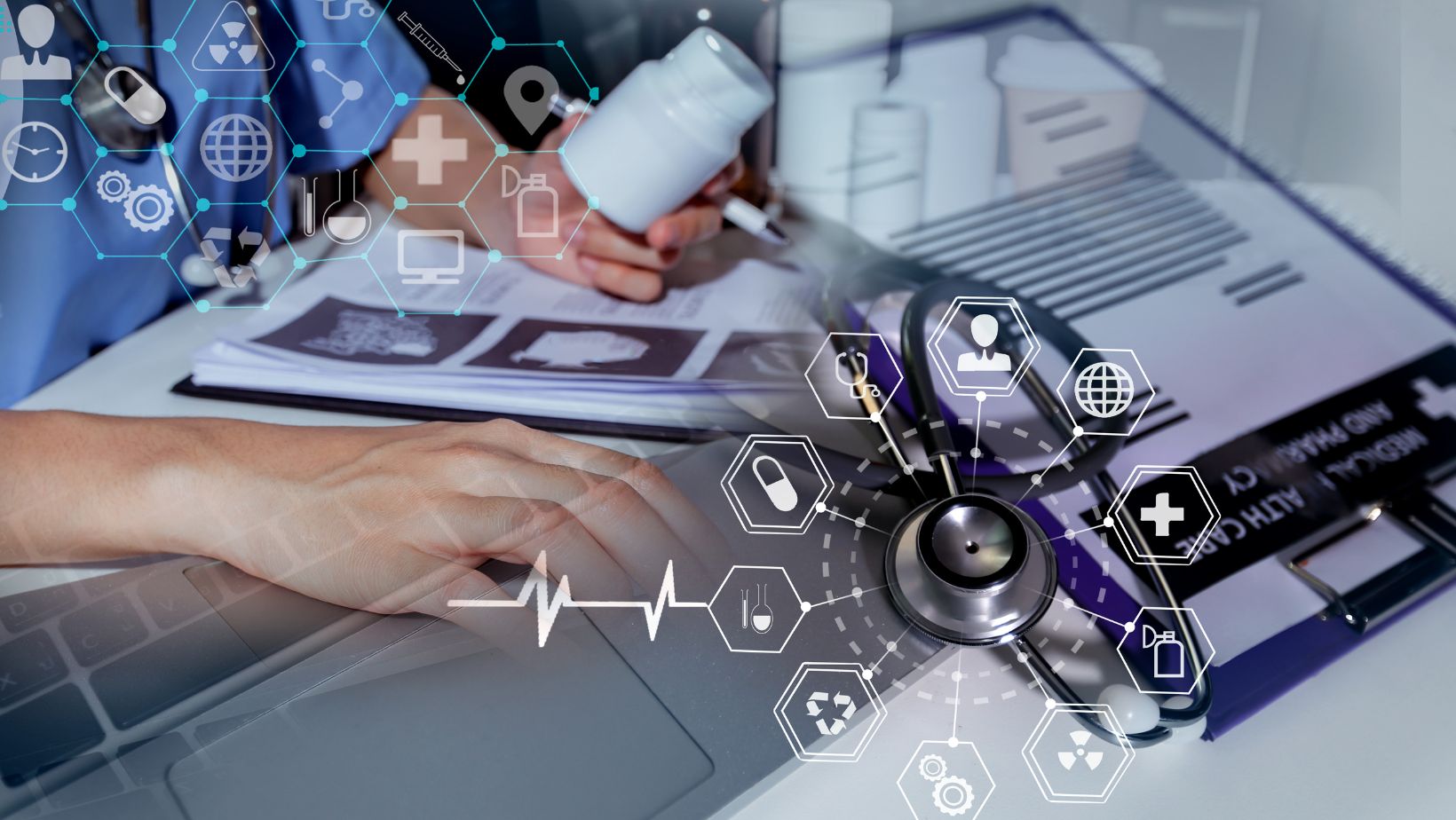
Data Security in Annotation
Key challenges include:
- Sensitive Data Protection: Medical records and personal images require strict security.
- Regulatory Compliance: Adhering to GDPR and CCPA is mandatory.
- Secure Collaboration: Encryption and access controls are vital for cloud-based platforms.
Investing in secure tools reduces risks and protects data assets.
Addressing Dataset Bias
Bias in annotations can lead to unfair AI outcomes. Ethical actions include:
- Diverse Representation: Avoid biased decision-making by ensuring dataset diversity.
- Fairness: Prevent overrepresentation of specific groups.
- Transparency: Document dataset creation and labeling processes.
Addressing bias promotes fairness and reliability in AI models.
Human Annotators and Ethics
Human annotators remain crucial for subjective tasks. Challenges involve:
- Fair Labor Practices: Ensure appropriate compensation and conditions.
- Avoiding Exploitation: Use transparent contracts in outsourcing.
- Training: Equip teams with tools and knowledge to meet standards.
Combining automation with human supervision maintains quality and upholds ethical standards.
What to Expect from Future Image Annotation Tools
Annotation tools are advancing to improve speed, accuracy, and scalability. Capabilities such as real-time collaboration, role-based permissions, and integrated communication enhance team efficiency. Tools now offer tailored workflows and support for complex data like 3D and video, making them adaptable to diverse needs.
AI-driven feedback, including error alerts and automated corrections, enhances efficiency. Automation features, such as AI-powered pre-annotations and seamless AI pipeline integration, reduce costs and save time while improving data quality.
Conclusion
The image annotation market is expanding quickly, fueled by AI advancements and rising data requirements. Businesses must adopt innovative tools, balance outsourcing and in-house strategies, and prioritize data security to stay competitive. Staying informed on trends ensures long-term success in this evolving field.